Automatic photo captioning is a problem where a model must generate a human-readable textual description given a photograph.
It is a challenging problem in artificial intelligence that requires both image understanding from the field of computer vision as well as language generation from the field of natural language processing.
It is now possible to develop your own image caption models using deep learning and freely available datasets of photos and their descriptions.
In this tutorial, you will discover how to prepare photos and textual descriptions ready for developing a deep learning automatic photo caption generation model.
After completing this tutorial, you will know:
- About the Flickr8K dataset comprised of more than 8,000 photos and up to 5 captions for each photo.
- How to generally load and prepare photo and text data for modeling with deep learning.
- How to specifically encode data for two different types of deep learning models in Keras.
Kick-start your project with my new book Deep Learning for Natural Language Processing, including step-by-step tutorials and the Python source code files for all examples.
Let’s get started.
- Update Nov/2017: Fixed small typos in the code in the “Whole Description Sequence Model” section. Thanks Moustapha Cheikh and Matthew.
- Update Feb/2019: Provided direct links for the Flickr8k_Dataset dataset, as the official site was taken down.
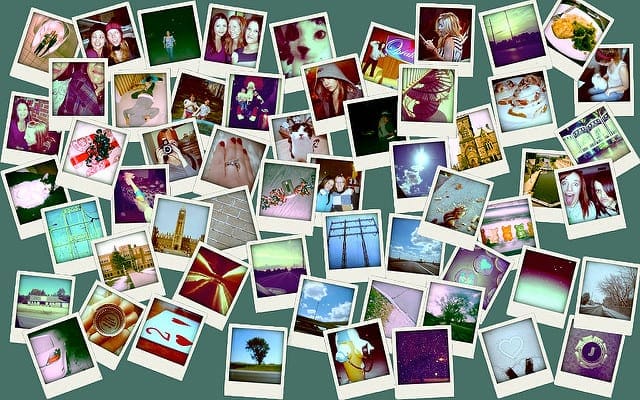
How to Prepare a Photo Caption Dataset for Training a Deep Learning Model
Photo by beverlyislike, some rights reserved.
Tutorial Overview
This tutorial is divided into 9 parts; they are:
- Download the Flickr8K Dataset
- How to Load Photographs
- Pre-Calculate Photo Features
- How to Load Descriptions
- Prepare Description Text
- Whole Description Sequence Model
- Word-By-Word Model
- Progressive Loading
- Pre-Calculate Photo Features
Python Environment
This tutorial assumes you have a Python 3 SciPy environment installed. You can use Python 2, but you may need to change some of the examples.
You must have Keras (2.0 or higher) installed with either the TensorFlow or Theano backend.
The tutorial also assumes you have scikit-learn, Pandas, NumPy and Matplotlib installed.
If you need help with your environment, see this post:
Need help with Deep Learning for Text Data?
Take my free 7-day email crash course now (with code).
Click to sign-up and also get a free PDF Ebook version of the course.
Download the Flickr8K Dataset
A good dataset to use when getting started with image captioning is the Flickr8K dataset.
The reason is that it is realistic and relatively small so that you can download it and build models on your workstation using a CPU.
The definitive description of the dataset is in the paper “Framing Image Description as a Ranking Task: Data, Models and Evaluation Metrics” from 2013.
The authors describe the dataset as follows:
We introduce a new benchmark collection for sentence-based image description and search, consisting of 8,000 images that are each paired with five different captions which provide clear descriptions of the salient entities and events.
…
The images were chosen from six different Flickr groups, and tend not to contain any well-known people or locations, but were manually selected to depict a variety of scenes and situations.
— Framing Image Description as a Ranking Task: Data, Models and Evaluation Metrics, 2013.
The dataset is available for free. You must complete a request form and the links to the dataset will be emailed to you. I would love to link to them for you, but the email address expressly requests: “Please do not redistribute the dataset“.
You can use the link below to request the dataset:
Within a short time, you will receive an email that contains links to two files:
- Flickr8k_Dataset.zip (1 Gigabyte) An archive of all photographs.
- Flickr8k_text.zip (2.2 Megabytes) An archive of all text descriptions for photographs.
UPDATE (Feb/2019): The official site seems to have been taken down (although the form still works). Here are some direct download links from my datasets GitHub repository:
Download the datasets and unzip them into your current working directory. You will have two directories:
- Flicker8k_Dataset: Contains 8092 photographs in jpeg format.
- Flickr8k_text: Contains a number of files containing different sources of descriptions for the photographs.
Next, let’s look at how to load the images.
How to Load Photographs
In this section, we will develop some code to load the photos for use with the Keras deep learning library in Python.
The image file names are unique image identifiers. For example, here is a sample of image file names:
Keras provides the load_img() function that can be used to load the image files directly as an array of pixels.
The pixel data needs to be converted to a NumPy array for use in Keras.
We can use the img_to_array() keras function to convert the loaded data.
We may want to use a pre-defined feature extraction model, such as a state-of-the-art deep image classification network trained on Image net. The Oxford Visual Geometry Group (VGG) model is popular for this purpose and is available in Keras.
The Oxford Visual Geometry Group (VGG) model is popular for this purpose and is available in Keras.
If we decide to use this pre-trained model as a feature extractor in our model, we can preprocess the pixel data for the model by using the preprocess_input() function in Keras, for example:
We may also want to force the loading of the photo to have the same pixel dimensions as the VGG model, which are 224 x 224 pixels. We can do that in the call to load_img(), for example:
We may want to extract the unique image identifier from the image filename. We can do that by splitting the filename string by the ‘.’ (period) character and retrieving the first element of the resulting array:
We can tie all of this together and develop a function that, given the name of the directory containing the photos, will load and pre-process all of the photos for the VGG model and return them in a dictionary keyed on their unique image identifiers.
Running this example prints the number of loaded images. It takes a few minutes to run.
If you do not have the RAM to hold all images (about 5GB by my estimation), then you can add an if-statement to break the loop early after 100 images have been loaded, for example:
Pre-Calculate Photo Features
It is possible to use a pre-trained model to extract the features from photos in the dataset and store the features to file.
This is an efficiency that means that the language part of the model that turns features extracted from the photo into textual descriptions can be trained standalone from the feature extraction model. The benefit is that the very large pre-trained models do not need to be loaded, held in memory, and used to process each photo while training the language model.
Later, the feature extraction model and language model can be put back together for making predictions on new photos.
In this section, we will extend the photo loading behavior developed in the previous section to load all photos, extract their features using a pre-trained VGG model, and store the extracted features to a new file that can be loaded and used to train the language model.
The first step is to load the VGG model. This model is provided directly in Keras and can be loaded as follows. Note that this will download the 500-megabyte model weights to your computer, which may take a few minutes.
This will load the VGG 16-layer model.
The two Dense output layers as well as the classification output layer are removed from the model by setting include_top=False. The output from the final pooling layer is taken as the features extracted from the image.
Next, we can walk over all images in the directory of images as in the previous section and call predict() function on the model for each prepared image to get the extracted features. The features can then be stored in a dictionary keyed on the image id.
The complete example is listed below.
The example may take some time to complete, perhaps one hour.
After all features are extracted, the dictionary is stored in the file ‘features.pkl‘ in the current working directory.
These features can then be loaded later and used as input for training a language model.
You could experiment with other types of pre-trained models in Keras.
How to Load Descriptions
It is important to take a moment to talk about the descriptions; there are a number available.
The file Flickr8k.token.txt contains a list of image identifiers (used in the image filenames) and tokenized descriptions. Each image has multiple descriptions.
Below is a sample of the descriptions from the file showing 5 different descriptions for a single image.
The file ExpertAnnotations.txt indicates which of the descriptions for each image were written by “experts” which were written by crowdsource workers asked to describe the image.
Finally, the file CrowdFlowerAnnotations.txt provides the frequency of crowd workers that indicate whether captions suit each image. These frequencies can be interpreted probabilistically.
The authors of the paper describe the annotations as follows:
… annotators were asked to write sentences that describe the depicted scenes, situations, events and entities (people, animals, other objects). We collected multiple captions for each image because there is a considerable degree of variance in the way many images can be described.
— Framing Image Description as a Ranking Task: Data, Models and Evaluation Metrics, 2013.
There are also lists of the photo identifiers to use in a train/test split so that you can compare results reported in the paper.
The first step is to decide which captions to use. The simplest approach is to use the first description for each photograph.
First, we need a function to load the entire annotations file (‘Flickr8k.token.txt‘) into memory. Below is a function to do this called load_doc() that, given a filename, will return the document as a string.
We can see from the sample of the file above that we need only split each line by white space and take the first element as the image identifier and the rest as the image description. For example:
We can then clean up the image identifier by removing the filename extension and the description number.
We can also put the description tokens back together into a string for later processing.
We can put all of this together into a function.
Below defines the load_descriptions() function that will take the loaded file, process it line-by-line, and return a dictionary of image identifiers to their first description.
Running the example prints the number of loaded image descriptions.
There are other ways to load descriptions that may turn out to be more accurate for the data.
Use the above example as a starting point and let me know what you come up with.
Post your approach in the comments below.
Prepare Description Text
The descriptions are tokenized; this means that each token is comprised of words separated by white space.
It also means that punctuation are separated as their own tokens, such as periods (‘.’) and apostrophes for word plurals (‘s).
It is a good idea to clean up the description text before using it in a model. Some ideas of data cleaning we can form include:
- Normalizing the case of all tokens to lowercase.
- Remove all punctuation from tokens.
- Removing all tokens that contain one or fewer characters (after punctuation is removed), e.g. ‘a’ and hanging ‘s’ characters.
We can implement these simple cleaning operations in a function that cleans each description in the loaded dictionary from the previous section. Below defines the clean_descriptions() function that will clean each loaded description.
We can then save the clean text to file for later use by our model.
Each line will contain the image identifier followed by the clean description. Below defines the save_doc() function for saving the cleaned descriptions to file.
Putting this all together with the loading of descriptions from the previous section, the complete example is listed below.
Running the example first loads 8,092 descriptions, cleans them, summarizes the vocabulary of 4,484 unique words, then saves them to a new file called ‘descriptions.txt‘.
Open the new file ‘descriptions.txt‘ in a text editor and review the contents. You should see somewhat readable descriptions of photos ready for modeling.
The vocabulary is still relatively large. To make modeling easier, especially the first time around, I would recommend further reducing the vocabulary by removing words that only appear once or twice across all descriptions.
Whole Description Sequence Model
There are many ways to model the caption generation problem.
One naive way is to create a model that outputs the entire textual description in a one-shot manner.
This is a naive model because it puts a heavy burden on the model to both interpret the meaning of the photograph and generate words, then arrange those words into the correct order.
This is not unlike the language translation problem used in an Encoder-Decoder recurrent neural network where the entire translated sentence is output one word at a time given an encoding of the input sequence. Here we would use an encoding of the image to generate the output sentence instead.
The image may be encoded using a pre-trained model used for image classification, such as the VGG trained on the ImageNet model mentioned above.
The output of the model would be a probability distribution over each word in the vocabulary. The sequence would be as long as the longest photo description.
The descriptions would, therefore, need to be first integer encoded where each word in the vocabulary is assigned a unique integer and sequences of words would be replaced with sequences of integers. The integer sequences would then need to be one hot encoded to represent the idealized probability distribution over the vocabulary for each word in the sequence.
We can use tools in Keras to prepare the descriptions for this type of model.
The first step is to load the mapping of image identifiers to clean descriptions stored in ‘descriptions.txt‘.
Running this piece loads the 8,092 photo descriptions into a dictionary keyed on image identifiers. These identifiers can then be used to load each photo file for the corresponding inputs to the model.
Next, we need to extract all of the description text so we can encode it.
We can use the Keras Tokenizer class to consistently map each word in the vocabulary to an integer. First, the object is created, then is fit on the description text. The fit tokenizer can later be saved to file for consistent decoding of the predictions back to vocabulary words.
Next, we can use the fit tokenizer to encode the photo descriptions into sequences of integers.
The model will require all output sequences to have the same length for training. We can achieve this by padding all encoded sequences to have the same length as the longest encoded sequence. We can pad the sequences with 0 values after the list of words. Keras provides the pad_sequences() function to pad the sequences.
Finally, we can one hot encode the padded sequences to have one sparse vector for each word in the sequence. Keras provides the to_categorical() function to perform this operation.
Once encoded, we can ensure that the sequence output data has the right shape for the model.
Putting all of this together, the complete example is listed below.
Running the example first prints the number of loaded image descriptions (8,092 photos), the dataset vocabulary size (4,485 words), the length of the longest description (28 words), then finally the shape of the data for fitting a prediction model in the form [samples, sequence length, features].
As mentioned, outputting the entire sequence may be challenging for the model.
We will look at a simpler model in the next section.
Word-By-Word Model
A simpler model for generating a caption for photographs is to generate one word given both the image as input and the last word generated.
This model would then have to be called recursively to generate each word in the description with previous predictions as input.
Using the word as input, give the model a forced context for predicting the next word in the sequence.
This is the model used in prior research, such as:
A word embedding layer can be used to represent the input words. Like the feature extraction model for the photos, this too can be pre-trained either on a large corpus or on the dataset of all descriptions.
The model would take a full sequence of words as input; the length of the sequence would be the maximum length of descriptions in the dataset.
The model must be started with something. One approach is to surround each photo description with special tags to signal the start and end of the description, such as ‘STARTDESC’ and ‘ENDDESC’.
For example, the description:
Would become:
And would be fed to the model with the same image input to result in the following input-output word sequence pairs:
The data preparation would begin much the same as was described in the previous section.
Each description must be integer encoded. After encoding, the sequences are split into multiple input and output pairs and only the output word (y) is one hot encoded. This is because the model is only required to predict the probability distribution of one word at a time.
The code is the same up to the point where we calculate the maximum length of sequences.
Next, we split the each integer encoded sequence into input and output pairs.
Let’s step through a single sequence called seq at the i’th word in the sequence, where i >= 1.
First, we take the first i-1 words as the input sequence and the i’th word as the output word.
Next, the input sequence is padded to the maximum length of the input sequences. Pre-padding is used (the default) so that new words appear at the end of the sequence, instead of the input beginning.
Pre-padding is used (the default) so that new words appear at the end of the sequence, instead of the beginning of the input.
The output word is one hot encoded, much like in the previous section.
We can put all of this together into a complete example to prepare description data for the word-by-word model.
Running the example prints the same statistics, but prints the size of the resulting encoded input and output sequences.
Note that the input of images must follow the exact same ordering where the same photo is shown for each example drawn from a single description. One way to do this would be to load the photo and store it for each example prepared from a single description.
Progressive Loading
The Flicr8K dataset of photos and descriptions can fit into RAM, if you have a lot of RAM (e.g. 8 Gigabytes or more), and most modern systems do.
This is fine if you want to fit a deep learning model using the CPU.
Alternately, if you want to fit a model using a GPU, then you will not be able to fit the data into memory of an average GPU video card.
One solution is to progressively load the photos and descriptions as-needed by the model.
Keras supports progressively loaded datasets by using the fit_generator() function on the model. A generator is the term used to describe a function used to return batches of samples for the model to train on. This can be as simple as a standalone function, the name of which is passed to the fit_generator() function when fitting the model.
As a reminder, a model is fit for multiple epochs, where one epoch is one pass through the entire training dataset, such as all photos. One epoch is comprised of multiple batches of examples where the model weights are updated at the end of each batch.
A generator must create and yield one batch of examples. For example, the average sentence length in the dataset is 11 words; that means that each photo will result in 11 examples for fitting the model and two photos will result in about 22 examples on average. A good default batch size for modern hardware may be 32 examples, so that is about 2-3 photos worth of examples.
We can write a custom generator to load a few photos and return the samples as a single batch.
Let’s assume we are working with a word-by-word model described in the previous section that expects a sequence of words and a prepared image as input and predicts a single word.
Let’s design a data generator that given a loaded dictionary of image identifiers to clean descriptions, a trained tokenizer, and a maximum sequence length will load one-image worth of examples for each batch.
A generator must loop forever and yield each batch of samples. If generators and yield are new concepts for you, consider reading this article:
We can loop forever with a while loop and within this, loop over each image in the image directory. For each image filename, we can load the image and create all of the input-output sequence pairs from the image’s description.
Below is the data generator function.
You could extend it to take the name of the dataset directory as a parameter.
The generator returns an array containing the inputs (X) and output (y) for the model. The input is comprised of an array with two items for the input images and encoded word sequences. The outputs are one hot encoded words.
You can see that it calls a function called load_photo() to load a single photo and return the pixels and image identifier. This is a simplified version of the photo loading function developed at the beginning of this tutorial.
Another function named create_sequences() is called to create sequences of images, input sequences of words, and output words that we then yield to the caller. This is a function that includes everything discussed in the previous section, and also creates copies of the image pixels, one for each input-output pair created from the photo’s description.
Prior to preparing the model that uses the data generator, we must load the clean descriptions, prepare the tokenizer, and calculate the maximum sequence length. All 3 of must be passed to the data_generator() as parameters.
We use the same load_clean_descriptions() function developed previously and a new create_tokenizer() function that simplifies the creation of the tokenizer.
Tying all of this together, the complete data generator is listed below, ready for use to train a model.
A data generator can be tested by calling the next() function.
We can test the generator as follows.
Running the example prints the shape of the input and output example for a single batch (e.g. 13 input-output pairs):
The generator can be used to fit a model by calling the fit_generator() function on the model (instead of fit()) and passing in the generator.
We must also specify the number of steps or batches per epoch. We could estimate this as (10 x training dataset size), perhaps 70,000 if 7,000 images are used for training.
Further Reading
This section provides more resources on the topic if you are looking go deeper.
Flickr8K Dataset
- Framing image description as a ranking task: data, models and evaluation metrics (Homepage)
- Framing Image Description as a Ranking Task: Data, Models and Evaluation Metrics, (PDF) 2013.
- Dataset Request Form
- Old Flicrk8K Homepage
API
Summary
In this tutorial, you discovered how to prepare photos and textual descriptions ready for developing an automatic photo caption generation model.
Specifically, you learned:
- About the Flickr8K dataset comprised of more than 8,000 photos and up to 5 captions for each photo.
- How to generally load and prepare photo and text data for modeling with deep learning.
- How to specifically encode data for two different types of deep learning models in Keras.
Do you have any questions?
Ask your questions in the comments below and I will do my best to answer.
No comments:
Post a Comment