Captioning an image involves generating a human readable textual description given an image, such as a photograph.
It is an easy problem for a human, but very challenging for a machine as it involves both understanding the content of an image and how to translate this understanding into natural language.
Recently, deep learning methods have displaced classical methods and are achieving state-of-the-art results for the problem of automatically generating descriptions, called “captions,” for images.
In this post, you will discover how deep neural network models can be used to automatically generate descriptions for images, such as photographs.
After completing this post, you will know:
- About the challenge of generating textual descriptions for images and the need to combine breakthroughs from computer vision and natural language processing.
- About the elements that comprise a neural feature captioning model, namely the feature extractor and language model.
- How the elements of the model can be arranged into an Encoder-Decoder, possibly with the use of an attention mechanism.
Kick-start your project with my new book Deep Learning for Natural Language Processing, including step-by-step tutorials and the Python source code files for all examples.
Let’s get started.
Overview
This post is divided into 3 parts; they are:
- Describing an Image with Text
- Neural Captioning Model
- Encoder-Decoder Architecture
Need help with Deep Learning for Text Data?
Take my free 7-day email crash course now (with code).
Click to sign-up and also get a free PDF Ebook version of the course.
Describing an Image with Text
Describing an image is the problem of generating a human-readable textual description of an image, such as a photograph of an object or scene.
The problem is sometimes called “automatic image annotation” or “image tagging.”
It is an easy problem for a human, but very challenging for a machine.
A quick glance at an image is sufficient for a human to point out and describe an immense amount of details about the visual scene. However, this remarkable ability has proven to be an elusive task for our visual recognition models
— Deep Visual-Semantic Alignments for Generating Image Descriptions, 2015.
A solution requires both that the content of the image be understood and translated to meaning in the terms of words, and that the words must string together to be comprehensible. It combines both computer vision and natural language processing and marks a true challenging problem in broader artificial intelligence.
Automatically describing the content of an image is a fundamental problem in artificial intelligence that connects computer vision and natural language processing.
— Show and Tell: A Neural Image Caption Generator, 2015.
Further, the problems can range in difficulty; let’s look at three different variations on the problem with examples.
1. Classify Image
Assign an image a class label from one of hundreds or thousands of known classes.
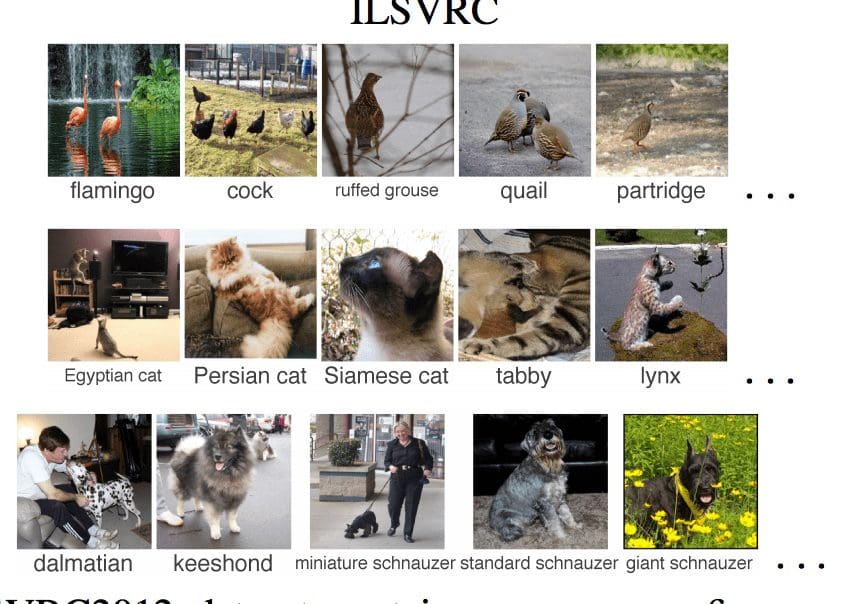
Example of classifying images into known classes
Taken From “Detecting avocados to zucchinis: what have we done, and where are we going?”, 2013.
2. Describe Image
Generate a textual description of the contents image.
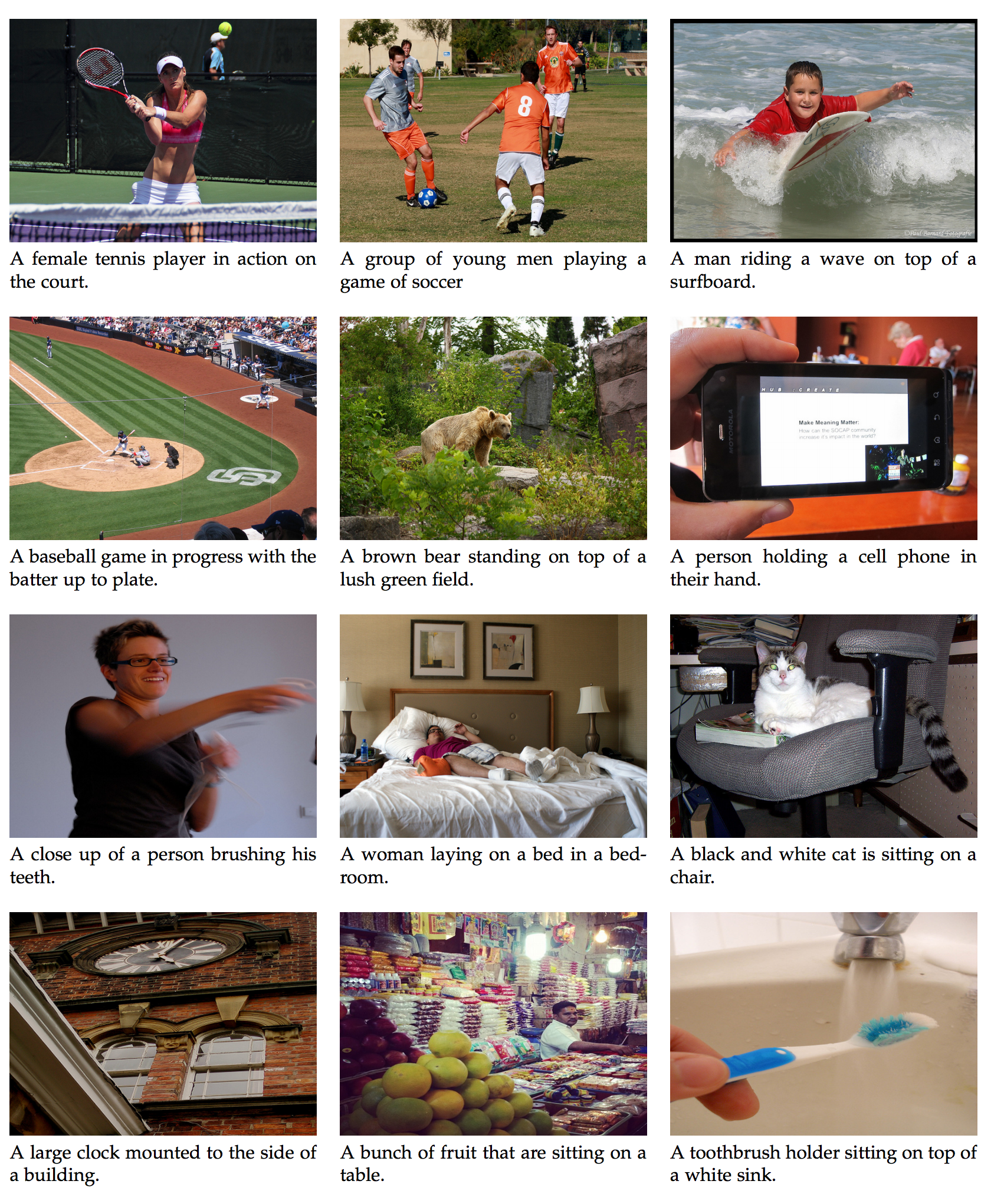
Example of captions generated for photogaphs
Taken from “Long-term recurrent convolutional networks for visual recognition and description”, 2015.
3. Annotate Image
Generate textual descriptions for specific regions on the image.
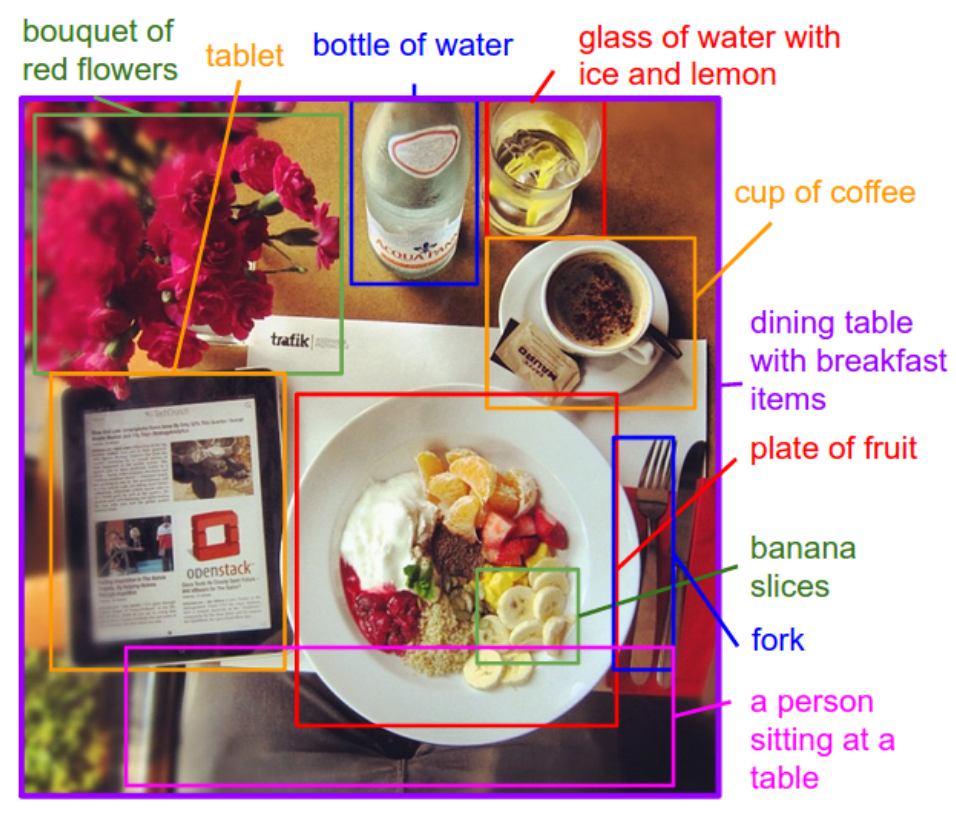
Example of annotation regions of an image with descriptions.
Taken from “Deep Visual-Semantic Alignments for Generating Image Descriptions”, 2015.
The general problem can also be extended to describe images over time in video.
In this post, we will focus our attention on describing images, which we will describe as ‘image captioning.’
Neural Captioning Model
Neural network models have come to dominate the field of automatic caption generation; this is primarily because the methods are demonstrating state-of-the-art results.
The two dominant methods prior to end-to-end neural network models for generating image captions were template-based methods and nearest-neighbor-based methods and modifying existing captions.
Prior to the use of neural networks for generating captions, two main approaches were dominant. The first involved generating caption templates which were filled in based on the results of object detections and attribute discovery. The second approach was based on first retrieving similar captioned images from a large database then modifying these retrieved captions to fit the query. […] Both of these approaches have since fallen out of favour to the now dominant neural network methods.
— Show, Attend and Tell: Neural Image Caption Generation with Visual Attention, 2015.
Neural network models for captioning involve two main elements:
- Feature Extraction.
- Language Model.
Feature Extraction Model
The feature extraction model is a neural network that given an image is able to extract the salient features, often in the form of a fixed-length vector.
The extracted features are an internal representation of the image, not something directly intelligible.
A deep convolutional neural network, or CNN, is used as the feature extraction submodel. This network can be trained directly on the images in the image captioning dataset.
Alternately, a pre-trained model, such as a state-of-the-art model used for image classification, can be used, or some hybrid where a pre-trained model is used and fine tuned on the problem.
It is popular to use top performing models in the ImageNet dataset developed for the ILSVRC challenge, such as the Oxford Vision Geometry Group model, called VGG for short.
[…] we explored several techniques to deal with overfitting. The most obvious way to not overfit is to initialize the weights of the CNN component of our system to a pretrained model (e.g., on ImageNet)
— Show, Attend and Tell: Neural Image Caption Generation with Visual Attention, 2015.
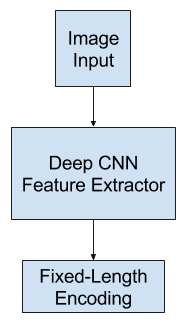
Feature Extractor
Language Model
Generally, a language model predicts the probability of the next word in the sequence given the words already present in the sequence.
For image captioning, the language model is a neural network that given the extracted features from the network is capable of predicting the sequence of words in the description and build up the description conditional on the words that have already been generated.
It is popular to use a recurrent neural network, such as a Long Short-Term Memory network, or LSTM, as the language model. Each output time step generates a new word in the sequence.
Each word that is generated is then encoded using a word embedding (such as word2vec) and passed as input to the decoder for generating the subsequent word.
An improvement to the model involves gathering the probability distribution of words across the vocabulary for the output sequence and searching it to generate multiple possible descriptions. These descriptions can be scored and ranked by likelihood. It is common to use a Beam Search for this search.
The language model can be trained standalone using pre-computed features extracted from the image dataset; it can be trained jointly with the feature extraction network, or some combination.
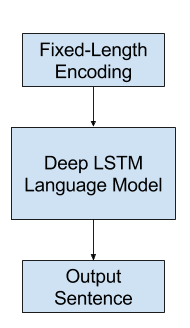
Language Model
Encoder-Decoder Architecture
A popular way to structure the sub-models is to use an Encoder-Decoder architecture where both models are trained jointly.
[the model] is based on a convolution neural network that encodes an image into a compact representation, followed by a recurrent neural network that generates a corresponding sentence. The model is trained to maximize the likelihood of the sentence given the image.
— Show and Tell: A Neural Image Caption Generator, 2015.
This is an architecture developed for machine translation where an input sequence, say in French, is encoded as a fixed-length vector by an encoder network. A separate decoder network then reads the encoding and generates an output sequence in the new language, say English.
A benefit of this approach in addition to the impressive skill of the approach is that a single end-to-end model can be trained on the problem.
When adapted for image captioning, the encoder network is a deep convolutional neural network, and the decoder network is a stack of LSTM layers.
[in machine translation] An “encoder” RNN reads the source sentence and transforms it into a rich fixed-length vector representation, which in turn in used as the initial hidden state of a “decoder” RNN that generates the target sentence. Here, we propose to follow this elegant recipe, replacing the encoder RNN by a deep convolution neural network (CNN).
— Show and Tell: A Neural Image Caption Generator, 2015.
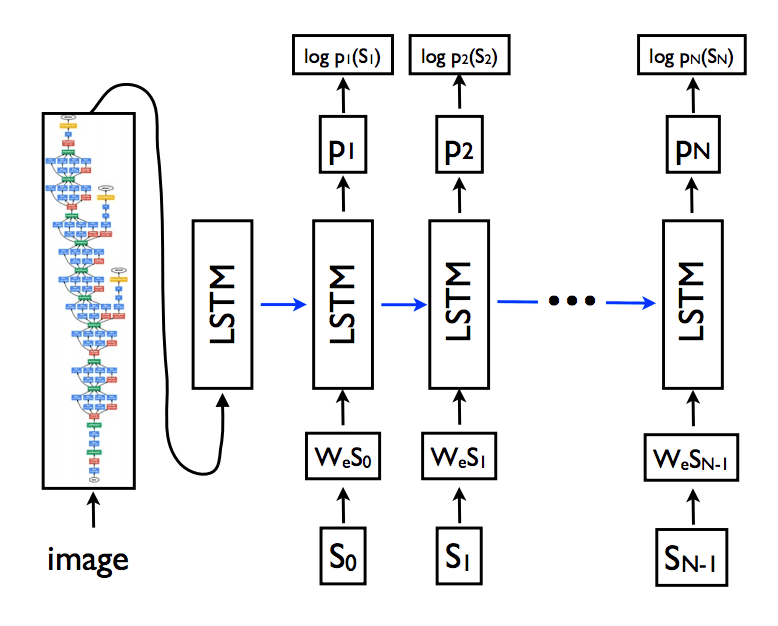
Example of the CNN and LSTM Architecture.
Taken from “Show and Tell: A Neural Image Caption Generator”, 2015.
Captioning Model with Attention
A limitation of the Encoder-Decoder architecture is that a single fixed-length representation is used to hold the extracted features.
This was addressed in machine translation through the development of attention across a richer encoding, allowing the decoder to learn where to place attention as each word in the translation is generated.
The approach of attention has also been used to improve the performance of the Encoder-Decoder architecture for image captioning by allowing the decoder to learn where to put attention in the image when generating each word in the description.
Encouraged by recent advances in caption generation and inspired by recent success in employing attention in machine translation and object recognition we investigate models that can attend to salient part of an image while generating its caption.
— Show, Attend and Tell: Neural Image Caption Generation with Visual Attention, 2015.
A benefit of this approach is that it is possible to visualize exactly where attention is placed while generating each word in a description.
We also show through visualization how the model is able to automatically learn to fix its gaze on salient objects while generating the corresponding words in the output sequence.
— Show, Attend and Tell: Neural Image Caption Generation with Visual Attention, 2015.
This is easiest to understand with an example; see below.
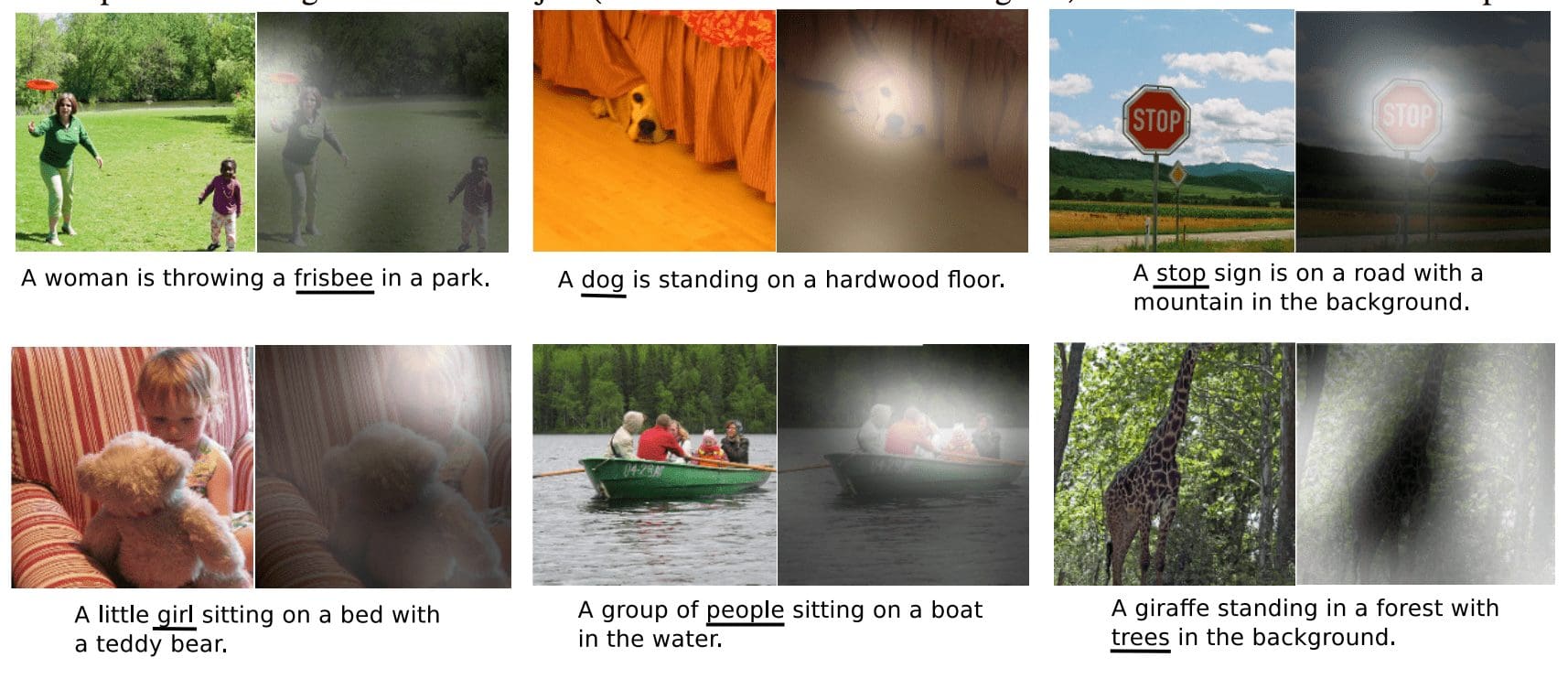
Example of image captioning with attention
Taken from “Show, Attend and Tell: Neural Image Caption Generation with Visual Attention”, 2015.
Further Reading
This section provides more resources on the topic if you are looking go deeper.
Papers
- Show and Tell: A Neural Image Caption Generator, 2015.
- Show, Attend and Tell: Neural Image Caption Generation with Visual Attention, 2015.
- Long-term recurrent convolutional networks for visual recognition and description, 2015.
- Deep Visual-Semantic Alignments for Generating Image Descriptions, 2015.
Articles
- Automatic image annotation on Wikipedia
- Show and Tell: image captioning open sourced in TensorFlow, 2016.
- Presentation: Automated Image Captioning with ConvNets and Recurrent Nets, Andrej Karpathy and Fei-Fei Li (slides).
Projects
- Project: Deep Visual-Semantic Alignments for Generating Image Descriptions, 2015.
- NeuralTalk2: Efficient Image Captioning code in Torch, runs on GPU, Andrej Karpathy.
Summary
In this post, you discovered how deep neural network models can be used to automatically generate descriptions for images, such as photographs.
Specifically, you learned:
- About the challenge of generating textual descriptions for images and the need to combine breakthroughs from computer vision and natural language processing.
- About the elements that comprise a neural feature captioning model, namely the feature extractor and language model.
- How the elements of the model can be arranged into an Encoder-Decoder, possibly with the use of an attention mechanism.
Do you have any questions?
Ask your questions in the comments below and I will do my best to answer.
No comments:
Post a Comment