ou’re interested in Machine Learning and maybe you dabble in it a little.
If you talk about Machine Learning with a friend or colleague one day, you run the risk of someone actually asking you:
“So, what is machine learning?“
The goal of this post is to give you a few definitions to think about and a handy one-liner definition that is easy to remember.
We will start out by getting a feeling for the standard definitions of Machine Learning taken from authoritative textbooks in the field. We’ll finish up by working out a developers definition of machine learning and a handy one-liner that we can use anytime we’re asked: What is Machine Learning?
Authoritative Definitions
Let’s start out by looking at four textbooks on Machine Learning that are commonly used in university-level courses.
These are our authoritative definitions and lay our foundation for deeper thought on the subject.
I chose these four definitions to highlight some useful and varied perspectives on the field. Through experience, we’ll learn that the field really is a mess of methods and choosing a perspective is key to making progress.
Mitchell’s Machine Learning
Tom Mitchell in his book Machine Learning provides a definition in the opening line of the preface:
The field of machine learning is concerned with the question of how to construct computer programs that automatically improve with experience.
I like this short and sweet definition and it is the basis for the developers definition we come up with at the end of the post.
Note the mention of “computer programs” and the reference to “automated improvement“. Write programs that improve themselves, it’s provocative!
In his introduction he provides a short formalism that you’ll see much repeated:
Don’t let the definition of terms scare you off, this is a very useful formalism.
We can use this formalism as a template and put E, T, and P at the top of columns in a table and list out complex problems with less ambiguity. It could be used as a design tool to help us think clearly about what data to collect (E), what decisions the software needs to make (T) and how we will evaluate its results (P). This power is why it is oft repeated as a standard definition. Keep it in your back pocket.
Elements of Statistical Learning
The Elements of Statistical Learning: Data Mining, Inference, and Prediction was written by three Stanford statisticians and self-described as a statistical framework to organize their field of inquiry.
In the preface is written:
Vast amounts of data are being generated in many fields, and the statisticians’s job is to make sense of it all: to extract important patterns and trends, and to understand “what the data says”. We call this learning from data.
I understand the job of a statistician is to use the tools of statistics to interpret data in the context of the domain. The authors seem to include all of the field of Machine Learning as aids in that pursuit. Interestingly, they chose to include “Data Mining” in the subtitle of the book.
Statisticians learn from data, but software does too and we learn from the things that the software learns. From the decisions made and the results achieved by various machine learning methods.
Pattern Recognition
Bishop in the preface of his book Pattern Recognition and Machine Learning comments:
Pattern recognition has its origins in engineering, whereas machine learning grew out of computer science. However, these activities can be viewed as two facets of the same field…
Reading this, you get the impression that Bishop came at the field from an engineering perspective and later learned and leveraged the Computer Science take on the same methods. Pattern recognition is an engineering or signal processing term.
This is a mature approach and one we should emulate. More broadly, regardless of the field that lays claim to a method, if it suits our needs by getting us closer to an insight or a result by “learning from data”, then we can decide to call it machine learning.
An Algorithmic Perspective
Marsland provides adopts the Mitchell definition of Machine Learning in his book Machine Learning: An Algorithmic Perspective.
He provides a cogent note in his prologue that motivates his writing the book:
One of the most interesting features of machine learning is that it lies on the boundary of several different academic disciplines, principally computer science, statistics, mathematics, and engineering. …machine learning is usually studied as part of artificial intelligence, which puts it firmly into computer science …understanding why these algorithms work requires a certain amount of statistical and mathematical sophistication that is often missing from computer science undergraduates.
This is insightful and instructive.
Firstly, he underscores the multidisciplinary nature of the field. We were getting a feeling for that from the above definition, but he draws a big red underline for us. Machine Learning draws from all manner of information sciences.
Secondly, he underscores the danger of sticking to a given perspective too tightly. Specifically, the case of a the algorithmist who shies away from the mathematical inner workings of a method.
No doubt, the counter case of the statistician that shies away from the practical concerns of implementation and deployment is just as limiting.
Venn Diagram
Drew Conway created a nice Venn Diagram in September 2010 that might help.
In his explanation, he comments: Machine Learning = Hacking + Math & Statistics
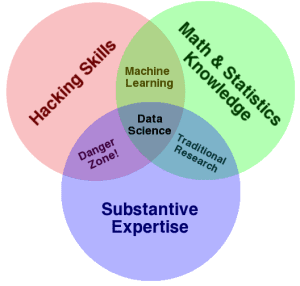
Data Science Venn Diagram. Credited to Drew Conway, Creative Commons licensed as Attribution-NonCommercial.
He also describes the Danger Zone as Hacking Skills + Expertise.
Here, he is referring to those people that know enough to be dangerous. They can access and structure data, they know the domain and they can run a method and present results, but don’t understand what the results mean. I think this is what Marsland may have been hinting at.
Developers Definition of Machine Learning
We now turn to the need to break all of this down to nuts and bolts for us developers.
We first look at complex problems that resist our decomposition and procedural solutions. This frames the power of machine learning. We then work out a definition that sits well with us developers that we can use whenever we’re asked, “So, What is Machine Learning?” by other developers.
Complex Problems
As a developer, you will eventually encounter classes of problems that stubbornly resist a logical and procedural solution.
What I mean is, there are classes of problems where it is not feasible or cost-effective to sit down and write out all the if statements needed to solve the problem.
“Sacrilege!” I hear your developer’s brain shout.
It’s true.
Take the every-day case of the decision problem of discriminating spam email from non-spam email. This is an example used all the time when introducing machine learning. How would you write a program to filter emails as they come into your email account and decide to put them in the spam folder or the inbox folder?
You’d probably start out by collecting some examples and having a look at them and a deep think about them. You’d look for patterns in the emails that are spam and those that are not. You’d think about abstracting those patterns so that your heuristics would work with new cases in the future. You’d ignore odd emails that will never be seen again. You’d go for easy wins to get your accuracy up and craft special things for the edge cases. You’d review the email frequently over time and think about abstracting new patterns to improve the decision making.
There’s a machine learning algorithm in there, amongst all that, except it was executed by you the programmer rather than the computer. This manually derived hardcoded system would only be as good as the programmer’s ability to extract rules from the data and implement them in the program.
It could be done, but it would take a lot of resources and be a maintenance nightmare.
Machine Learning
In the example above, I’m sure your developer brain, that part of your brain that ruthlessly seeks to automate, could see the opportunity for automating and optimizing the meta-process of extracting patterns from examples.
Machine learning methods are this automated process.
In our spam/non-spam example, the examples (E) are emails we have collected. The task (T) was a decision problem (called classification) of marking each email as spam or not, and putting it in the correct folder. Our performance measure (P) would be something like accuracy as a percentage (correct decisions divided by total decisions made multiplied by 100) between 0% (worst) and 100% (best).
Preparing a decision making program like this is typically called training, where collected examples are called the training set and the program is referred to as a model, as in a model of the problem of classifying spam from non-spam. As developers, we like this terminology, a model has state and needs to be persisted, training is a process that is performed once and is maybe rerun as needed, classification is the task performed. It all makes sense to us.
We can see that some of the terminology used in the above definitions does not sit well for programmers. Technically, all the programs we write are automations, commenting that machine learning automatically learns is not meaningful.
Handy One-liner
So, let’s see if we can use these pieces and construct a developers definition of machine learning. How about:
Machine Learning is the training of a model from data that generalizes a decision against a performance measure.
Training a model suggests training examples. A model suggests state acquired through experience. Generalizes a decision suggests the capability to make a decision based on inputs and anticipating unseen inputs in the future for which a decision will be required. Finally, against a performance measure suggests a targeted need and directed quality to the model being prepared.
I’m no poet, can you come up with a more accurate or more succinct developers definition of Machine Learning?
Share your definition in the comments below.
Further Reading
I’ve linked to resources throughout this post, but I have listed some useful resources below if you thirst for more reading.
Books
The following are the four textbooks from which definitions were drawn.
- Machine Learning by Mitchell
- The Elements of Statistical Learning: Data Mining, Inference, and Prediction by Hastie, Tibshirani and Friedman
- Pattern Recognition and Machine Learning by Bishop
- Machine Learning: An Algorithmic Perspective by Marsland.
Also, Drew Conway has a book in collaboration with John Myles White that is practical and fun to read titled Machine Learning for Hackers.
Question and Answer Sites
There are some interesting discussions on Q&A websites about what exactly machine learning is, below are some picks.
- Quora is well suited to high-level questions like this, have a browse through some. My picks are: What is machine learning in layman’s terms? and What is data science?
- Cross Validated has some great discussions on this higher-level question. See The Two Cultures: statistics vs. machine learning? Two resources mentioned in this discussion are the blog post Statistics vs. Machine Learning, fight! and the paper Statistical Modeling: The Two Cultures.
- Stack Overflow also has some discussion, for example, checkout What is machine learning?
I’ve thought hard about all of this, and my definition is coloured by the books I’ve read and the experiences I’ve had. Let me know if it’s useful.
Leave a comment and let us all know how you understand the field.
What is Machine Learning to you? Do you know of any further resources we
could fall back to?
Let me know in the comments below.
No comments:
Post a Comment