Particle swarm optimization (PSO) is one of the bio-inspired algorithms and it is a simple one to search for an optimal solution in the solution space. It is different from other optimization algorithms in such a way that only the objective function is needed and it is not dependent on the gradient or any differential form of the objective. It also has very few hyperparameters.
In this tutorial, you will learn the rationale of PSO and its algorithm with an example. After competing this tutorial, you will know:
- What is a particle swarm and their behavior under the PSO algorithm
- What kind of optimization problems can be solved by PSO
- How to solve a problem using particle swarm optimization
- What are the variations of the PSO algorithm
Kick-start your project with my new book Optimization for Machine Learning, including step-by-step tutorials and the Python source code files for all examples.
Let’s get started.Particle Swarms
Particle Swarm Optimization was proposed by Kennedy and Eberhart in 1995. As mentioned in the original paper, sociobiologists believe a school of fish or a flock of birds that moves in a group “can profit from the experience of all other members”. In other words, while a bird flying and searching randomly for food, for instance, all birds in the flock can share their discovery and help the entire flock get the best hunt.
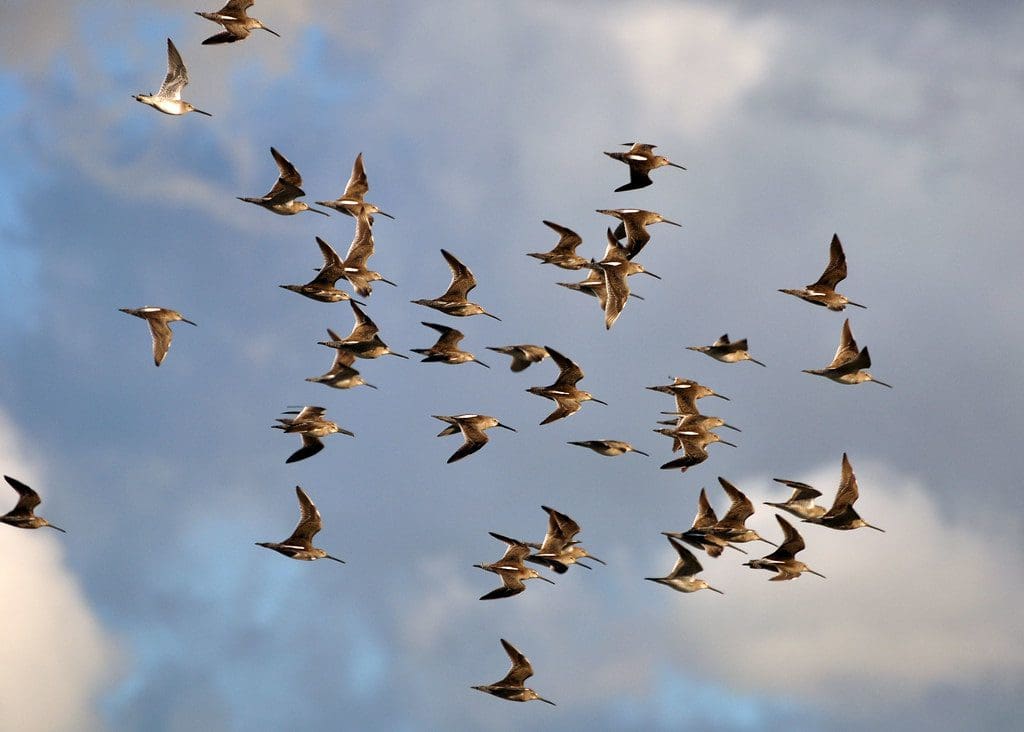
Particle Swarm Optimizsation.
Photo by Don DeBold, some rights reserved.
While we can simulate the movement of a flock of birds, we can also imagine each bird is to help us find the optimal solution in a high-dimensional solution space and the best solution found by the flock is the best solution in the space. This is a heuristic solution because we can never prove the real global optimal solution can be found and it is usually not. However, we often find that the solution found by PSO is quite close to the global optimal.
Example Optimization Problem
PSO is best used to find the maximum or minimum of a function defined on a multidimensional vector space. Assume we have a function
Let’s start with the following function
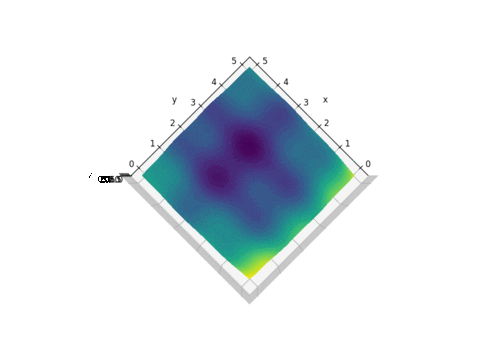
Plot of f(x,y)
As we can see from the plot above, this function looks like a curved egg carton. It is not a convex function and therefore it is hard to find its minimum because a local minimum found is not necessarily the global minimum.
So how can we find the minimum point in this function? For sure, we can resort to exhaustive search: If we check the value of
This is how a particle swarm optimization does. Similar to the flock of birds looking for food, we start with a number of random points on the plane (call them particles) and let them look for the minimum point in random directions. At each step, every particle should search around the minimum point it ever found as well as around the minimum point found by the entire swarm of particles. After certain iterations, we consider the minimum point of the function as the minimum point ever explored by this swarm of particles.
Want to Get Started With Optimization Algorithms?
Take my free 7-day email crash course now (with sample code).
Click to sign-up and also get a free PDF Ebook version of the course.
Algorithm Details
Assume we have
or, equivalently,
and at the same time, the velocities are also updated by the rule
where
Note that
We call the parameter
The positions
One interesting property of this algorithm that distinguishs it from other optimization algorithms is that it does not depend on the gradient of the objective function. In gradient descent, for example, we look for the minimum of a function
Another property of PSO is that it can be parallelized easily. As we are manipulating multiple particles to find the optimal solution, each particles can be updated in parallel and we only need to collect the updated value of
Implementation
Here we show how we can implement PSO to find the optimal solution.
For the same function as we showed above, we can first define it as a Python function and show it in a contour plot:
1 2 3 4 5 6 7 8 9 10 11 12 13 14 15 16 17 18 19 | import numpy as np import matplotlib.pyplot as plt def f(x,y): "Objective function" return (x-3.14)**2 + (y-2.72)**2 + np.sin(3*x+1.41) + np.sin(4*y-1.73) # Contour plot: With the global minimum showed as "X" on the plot x, y = np.array(np.meshgrid(np.linspace(0,5,100), np.linspace(0,5,100))) z = f(x, y) x_min = x.ravel()[z.argmin()] y_min = y.ravel()[z.argmin()] plt.figure(figsize=(8,6)) plt.imshow(z, extent=[0, 5, 0, 5], origin='lower', cmap='viridis', alpha=0.5) plt.colorbar() plt.plot([x_min], [y_min], marker='x', markersize=5, color="white") contours = plt.contour(x, y, z, 10, colors='black', alpha=0.4) plt.clabel(contours, inline=True, fontsize=8, fmt="%.0f") plt.show() |
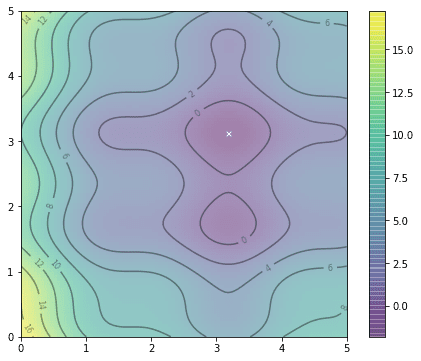
Contour plot of f(x,y)
Here we plotted the function
1 2 3 | n_particles = 20 X = np.random.rand(2, n_particles) * 5 V = np.random.randn(2, n_particles) * 0.1 |
which we can show their position on the same contour plot:
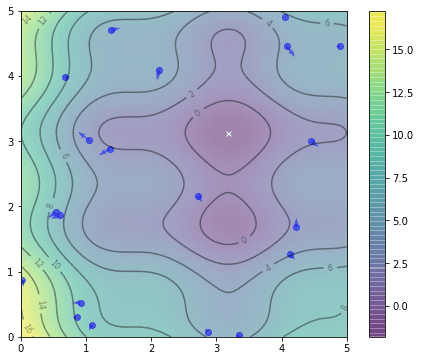
Initial position of particles
From this, we can already find the
1 2 3 4 | pbest = X pbest_obj = f(X[0], X[1]) gbest = pbest[:, pbest_obj.argmin()] gbest_obj = pbest_obj.min() |
The vector pbest_obj
is the best value of the objective function found by each particle. Similarly, gbest_obj
is the best scalar value of the objective function ever found by the swarm. We are using min()
and argmin()
functions here because we set it as a minimization problem. The position of gbest
is marked as a star below
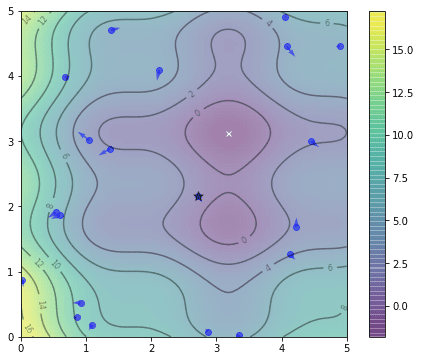
Position of gbest is marked as a star
Let’s set
1 2 3 4 5 6 7 8 9 10 11 | c1 = c2 = 0.1 w = 0.8 # One iteration r = np.random.rand(2) V = w * V + c1*r[0]*(pbest - X) + c2*r[1]*(gbest.reshape(-1,1)-X) X = X + V obj = f(X[0], X[1]) pbest[:, (pbest_obj >= obj)] = X[:, (pbest_obj >= obj)] pbest_obj = np.array([pbest_obj, obj]).max(axis=0) gbest = pbest[:, pbest_obj.argmin()] gbest_obj = pbest_obj.min() |
The following is the position after the first iteration. We mark the best position of each particle with a black dot to distinguish from their current position, which are set in blue.
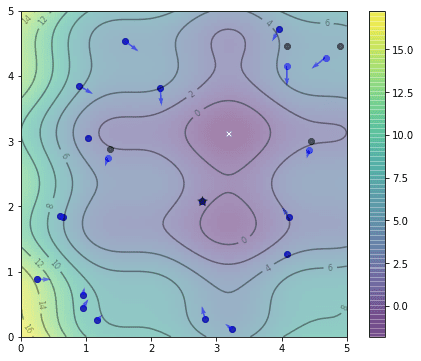
Positions of particles after one iteration
We can repeat the above code segment for multiple times and see how the particles explore. This is the result after the second iteration:
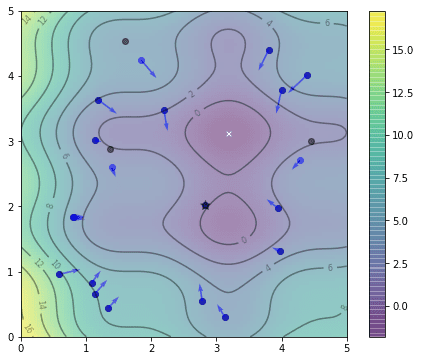
Positions of particles after two iterations
and this is after the 5th iteration, note that the position of
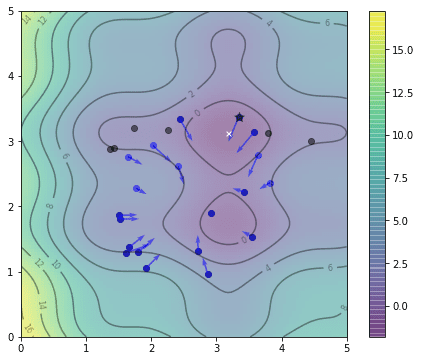
Positions of particles after 5 iterations
and after 20th iteration, we already very close to the optimal:
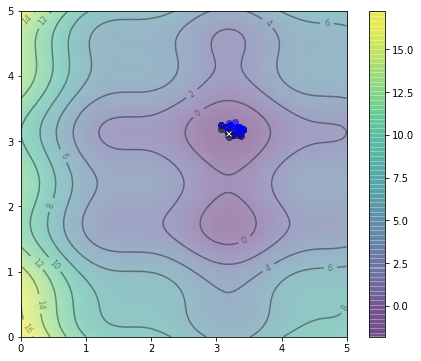
Positions of particles after 20 iterations
This is the animation showing how we find the optimal solution as the algorithm progressed. See if you may find some resemblance to the movement of a flock of birds:
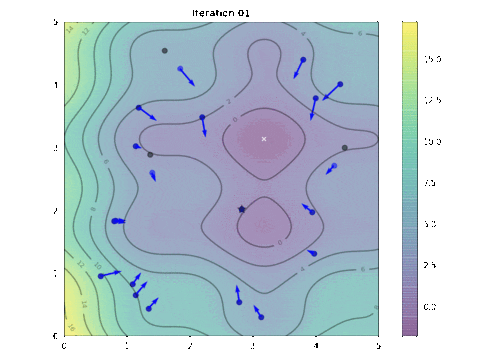
Animation of particle movements
So how close is our solution? In this particular example, the global minimum we found by exhaustive search is at the coordinate
Variations
All PSO algorithms are mostly the same as we mentioned above. In the above example, we set the PSO to run in a fixed number of iterations. It is trivial to set the number of iterations to run dynamically in response to the progress. For example, we can make it stop once we cannot see any update to the global best solution
Research on PSO were mostly on how to determine the hyperparameters
Complete Example
It should be easy to see how we can change the above code to solve a higher dimensional objective function, or switching from minimization to maximization. The following is the complete example of finding the minimum point of the function
1 2 3 4 5 6 7 8 9 10 11 12 13 14 15 16 17 18 19 20 21 22 23 24 25 26 27 28 29 30 31 32 33 34 35 36 37 38 39 40 41 42 43 44 45 46 47 48 49 50 51 52 53 54 55 56 57 58 59 60 61 62 63 64 65 66 67 68 69 70 71 72 73 74 75 76 77 78 79 | import numpy as np import matplotlib.pyplot as plt from matplotlib.animation import FuncAnimation def f(x,y): "Objective function" return (x-3.14)**2 + (y-2.72)**2 + np.sin(3*x+1.41) + np.sin(4*y-1.73) # Compute and plot the function in 3D within [0,5]x[0,5] x, y = np.array(np.meshgrid(np.linspace(0,5,100), np.linspace(0,5,100))) z = f(x, y) # Find the global minimum x_min = x.ravel()[z.argmin()] y_min = y.ravel()[z.argmin()] # Hyper-parameter of the algorithm c1 = c2 = 0.1 w = 0.8 # Create particles n_particles = 20 np.random.seed(100) X = np.random.rand(2, n_particles) * 5 V = np.random.randn(2, n_particles) * 0.1 # Initialize data pbest = X pbest_obj = f(X[0], X[1]) gbest = pbest[:, pbest_obj.argmin()] gbest_obj = pbest_obj.min() def update(): "Function to do one iteration of particle swarm optimization" global V, X, pbest, pbest_obj, gbest, gbest_obj # Update params r1, r2 = np.random.rand(2) V = w * V + c1*r1*(pbest - X) + c2*r2*(gbest.reshape(-1,1)-X) X = X + V obj = f(X[0], X[1]) pbest[:, (pbest_obj >= obj)] = X[:, (pbest_obj >= obj)] pbest_obj = np.array([pbest_obj, obj]).min(axis=0) gbest = pbest[:, pbest_obj.argmin()] gbest_obj = pbest_obj.min() # Set up base figure: The contour map fig, ax = plt.subplots(figsize=(8,6)) fig.set_tight_layout(True) img = ax.imshow(z, extent=[0, 5, 0, 5], origin='lower', cmap='viridis', alpha=0.5) fig.colorbar(img, ax=ax) ax.plot([x_min], [y_min], marker='x', markersize=5, color="white") contours = ax.contour(x, y, z, 10, colors='black', alpha=0.4) ax.clabel(contours, inline=True, fontsize=8, fmt="%.0f") pbest_plot = ax.scatter(pbest[0], pbest[1], marker='o', color='black', alpha=0.5) p_plot = ax.scatter(X[0], X[1], marker='o', color='blue', alpha=0.5) p_arrow = ax.quiver(X[0], X[1], V[0], V[1], color='blue', width=0.005, angles='xy', scale_units='xy', scale=1) gbest_plot = plt.scatter([gbest[0]], [gbest[1]], marker='*', s=100, color='black', alpha=0.4) ax.set_xlim([0,5]) ax.set_ylim([0,5]) def animate(i): "Steps of PSO: algorithm update and show in plot" title = 'Iteration {:02d}'.format(i) # Update params update() # Set picture ax.set_title(title) pbest_plot.set_offsets(pbest.T) p_plot.set_offsets(X.T) p_arrow.set_offsets(X.T) p_arrow.set_UVC(V[0], V[1]) gbest_plot.set_offsets(gbest.reshape(1,-1)) return ax, pbest_plot, p_plot, p_arrow, gbest_plot anim = FuncAnimation(fig, animate, frames=list(range(1,50)), interval=500, blit=False, repeat=True) anim.save("PSO.gif", dpi=120, writer="imagemagick") print("PSO found best solution at f({})={}".format(gbest, gbest_obj)) print("Global optimal at f({})={}".format([x_min,y_min], f(x_min,y_min))) |
Further Reading
These are the original papers that proposed the particle swarm optimization, and the early research on refining its hyperparameters:
- Kennedy J. and Eberhart R. C. Particle swarm optimization. In Proceedings of the International Conference on Neural Networks; Institute of Electrical and Electronics Engineers. Vol. 4. 1995. pp. 1942–1948. DOI: 10.1109/ICNN.1995.488968
- Eberhart R. C. and Shi Y. Comparing inertia weights and constriction factors in particle swarm optimization. In Proceedings of the 2000 Congress on Evolutionary Computation (CEC ‘00). Vol. 1. 2000. pp. 84–88. DOI: 10.1109/CEC.2000.870279
- Shi Y. and Eberhart R. A modified particle swarm optimizer. In Proceedings of the IEEE International Conferences on Evolutionary Computation, 1998. pp. 69–73. DOI: 10.1109/ICEC.1998.699146
Summary
In this tutorial we learned:
- How particle swarm optimization works
- How to implement the PSO algorithm
- Some possible variations in the algorithm
As particle swarm optimization does not have a lot of hyper-parameters and very permissive on the objective function, it can be used to solve a wide range of problems.
No comments:
Post a Comment