You do not need to be a Python developer to get started using the Python ecosystem for machine learning.
As a developer who already knows how to program in one or more programming languages, you are able to pick up a new language like Python very quickly. You just need to know a few properties of the language to transfer what you already know to the new language.
In this post, you will get a crash course in Python and the core libraries needed for machine learning. Namely: NumPy, MatPlotLib and Pandas.
This will be just enough information to help you read and understand code Python code examples for machine learning and start developing your own scripts. If you already know a little Python, this post will be a friendly reminder for you.
Python Crash Course
When getting started in Python you need to know a few key details about the language syntax to be able to read and understand Python code. This includes:
- Assignment
- Flow Control
- Data Structures
- Functions
We will cover each of these topics in turn with small standalone examples that you can type and run.
Remember, whitespace has meaning in Python.
Need help with Machine Learning in Python?
Take my free 2-week email course and discover data prep, algorithms and more (with code).
Click to sign-up now and also get a free PDF Ebook version of the course.
Assignment
As a programmer, assignment and types should not be surprising to you.
Strings
# Strings data = 'hello world' print(data[0]) print(len(data)) print(data) |
Running the example prints:
h 11 hello world |
Numbers
# Numbers value = 123.1 print(value) value = 10 print(value) |
Running the example prints:
123.1 10 |
Boolean
# Boolean a = True b = False print(a, b) |
Running the example prints:
(True, False) |
Multiple Assignment
# Multiple Assignment a, b, c = 1, 2, 3 print(a, b, c) |
Running the example prints:
(1, 2, 3) |
No Value
# No value a = None print(a) |
Running the example prints:
None |
Flow Control
There are three main types of flow control that you need to learn: If-Then-Else conditions, For-Loops and While-Loops.
If-Then-Else Condition Example
value = 99 if value >= 99: print('That is fast') elif value > 200: print('That is too fast') else: print('That that is safe') |
Running this example prints:
That is fast |
For-Loop Example
# For-Loop for i in range(10): print(i) |
Running this example prints:
0 1 2 3 4 5 6 7 8 9 |
While-Loop Example
# While-Loop i = 0 while i < 10: print(i) i += 1 |
Running this example prints:
0 1 2 3 4 5 6 7 8 9 |
Data Structures
There are three data structures in Python that you will find the most used and useful. They are tuples, lists and dictionaries.
Tuple Example
Tuples are read-only collections of items.
a = (1, 2, 3) print(a) |
Running the example prints:
(1, 2, 3) |
List Example
Lists use the square bracket notation and can be index using array notation.
mylist = [1, 2, 3] print("Zeroth Value: %d" % mylist[0]) mylist.append(4) print("List Length: %d" % len(mylist)) for value in mylist: print(value) |
Running the example prints:
Zeroth Value: 1 List Length: 4 1 2 3 4 |
Dictionary Example
Dictionaries are mappings of names to values, like a map. Note the use of the curly bracket notation.
mydict = {'a': 1, 'b': 2, 'c': 3} print("A value: %d" % mydict['a']) mydict['a'] = 11 print("A value: %d" % mydict['a']) print("Keys: %s" % mydict.keys()) print("Values: %s" % mydict.values()) for key in mydict.keys(): print(mydict[key]) |
Running the example prints:
A value: 1 A value: 11 Keys: ['a', 'c', 'b'] Values: [11, 3, 2] 11 3 2 |
Functions
The biggest gotcha with Python is the whitespace. Ensure that you have an empty new line after indented code.
The example below defines a new function to calculate the sum of two values and calls the function with two arguments.
# Sum function def mysum(x, y): return x + y # Test sum function print(mysum(1, 3)) |
Running the example prints:
4 |
NumPy Crash Course
NumPy provides the foundation data structures and operations for SciPy. These are arrays (ndarrays) that are efficient to define and manipulate.
Create Array
# define an array import numpy mylist = [1, 2, 3] myarray = numpy.array(mylist) print(myarray) print(myarray.shape) |
Running the example prints:
[1 2 3] (3,) |
Access Data
Array notation and ranges can be used to efficiently access data in a NumPy array.
# access values import numpy mylist = [[1, 2, 3], [3, 4, 5]] myarray = numpy.array(mylist) print(myarray) print(myarray.shape) print("First row: %s" % myarray[0]) print("Last row: %s" % myarray[-1]) print("Specific row and col: %s" % myarray[0, 2]) print("Whole col: %s" % myarray[:, 2]) |
Running the example prints:
[[1 2 3] [3 4 5]] (2, 3) First row: [1 2 3] Last row: [3 4 5] Specific row and col: 3 Whole col: [3 5] |
Arithmetic
NumPy arrays can be used directly in arithmetic.
# arithmetic import numpy myarray1 = numpy.array([2, 2, 2]) myarray2 = numpy.array([3, 3, 3]) print("Addition: %s" % (myarray1 + myarray2)) print("Multiplication: %s" % (myarray1 * myarray2)) |
Running the example prints:
Addition: [5 5 5] Multiplication: [6 6 6] |
There is a lot more to NumPy arrays but these examples give you a flavor of the efficiencies they provide when working with lots of numerical data.
Matplotlib Crash Course
Matplotlib can be used for creating plots and charts.
The library is generally used as follows:
- Call a plotting function with some data (e.g. plot()).
- Call many functions to setup the properties of the plot (e.g. labels and colors).
- Make the plot visible (e.g. show()).
Line Plot
The example below creates a simple line plot from one-dimensional data.
# basic line plot import matplotlib.pyplot as plt import numpy myarray = numpy.array([1, 2, 3]) plt.plot(myarray) plt.xlabel('some x axis') plt.ylabel('some y axis') plt.show() |
Running the example produces:
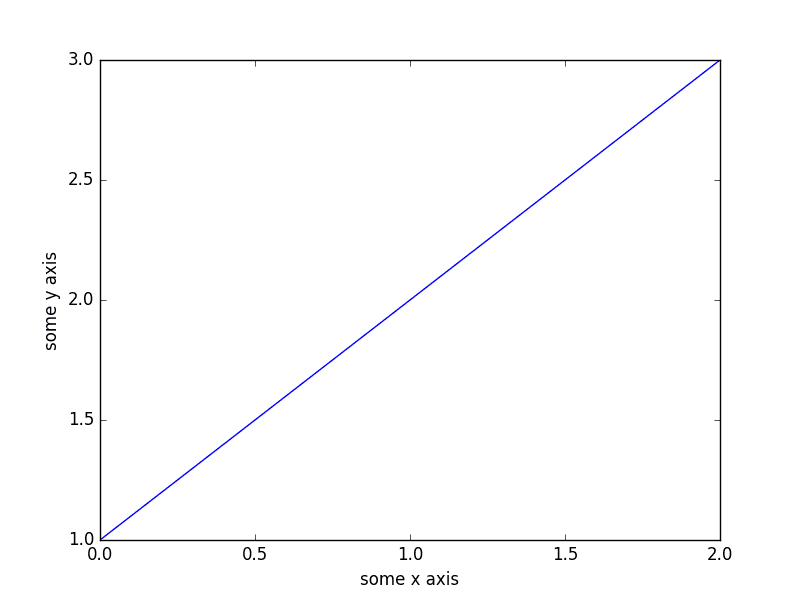
Simple Line Plot in Matplotlib
Scatter Plot
Below is a simple example of creating a scatter plot from two-dimensional data.
# basic scatter plot import matplotlib.pyplot as plt import numpy x = numpy.array([1, 2, 3]) y = numpy.array([2, 4, 6]) plt.scatter(x,y) plt.xlabel('some x axis') plt.ylabel('some y axis') plt.show() |
Running the example produces:
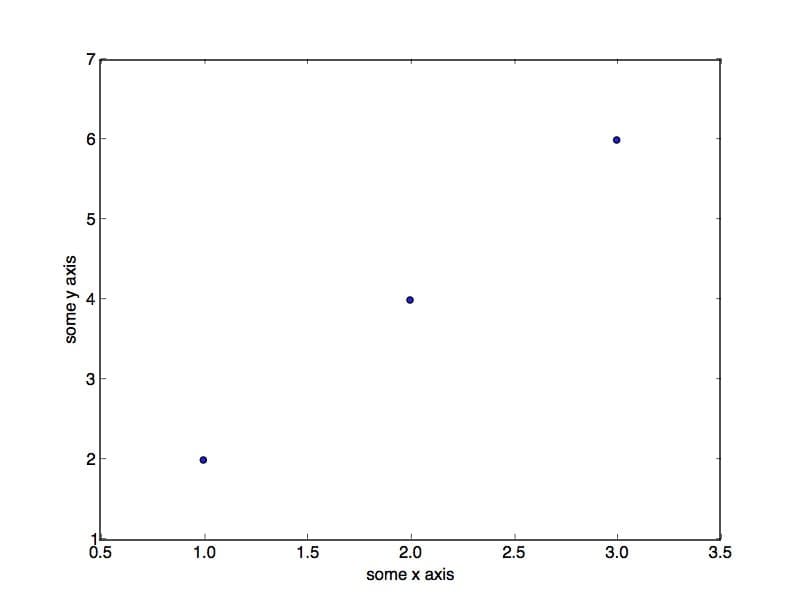
Simple Scatter Plot in Matplotlib
There are many more plot types and many more properties that can be set on a plot to configure it.
Pandas Crash Course
Pandas provides data structures and functionality to quickly manipulate and analyze data. The key to understanding Pandas for machine learning is understanding the Series and DataFrame data structures.
Series
A series is a one-dimensional array where the rows and columns can be labeled.
# series import numpy import pandas myarray = numpy.array([1, 2, 3]) rownames = ['a', 'b', 'c'] myseries = pandas.Series(myarray, index=rownames) print(myseries) |
Running the example prints:
a 1 b 2 c 3 |
You can access the data in a series like a NumPy array and like dictionary, for example:
print(myseries[0]) print(myseries['a']) |
Running the example prints:
1 1 |
DataFrame
A data frame is a multi-dimensional array where the rows and the columns can be labeled.
# dataframe import numpy import pandas myarray = numpy.array([[1, 2, 3], [4, 5, 6]]) rownames = ['a', 'b'] colnames = ['one', 'two', 'three'] mydataframe = pandas.DataFrame(myarray, index=rownames, columns=colnames) print(mydataframe) |
Running the example prints:
one two three a 1 2 3 b 4 5 6 |
Data can be index using column names.
print("one column: %s" % mydataframe['one']) print("one column: %s" % mydataframe.one) |
Running the example prints:
one column: a 1 b 4 one column: a 1 b 4 |
Summary
You have covered a lot of ground in this post. You discovered basic syntax and usage of Python and four key Python libraries used for machine learning:
- NumPy
- Matplotlib
- Pandas
You now know enough syntax and usage information to read and understand Python code for machine learning and to start creating your own scripts.
Do you have any questions about the examples in this post? Ask your questions in the comments and I will do my best to answer.
No comments:
Post a Comment